It’s likely you’ve run into this. You’ve created a machine learning (ML) model and trained it with plenty of data. Validation results look very promising, so you ship the model to pre-production and suddenly something’s not right. The model hasn’t failed outright, but your metrics do not align with what you observed in the training and evaluation phase. It’s time to block out time to do a sanity check so you can figure out what went wrong and how to fix it.
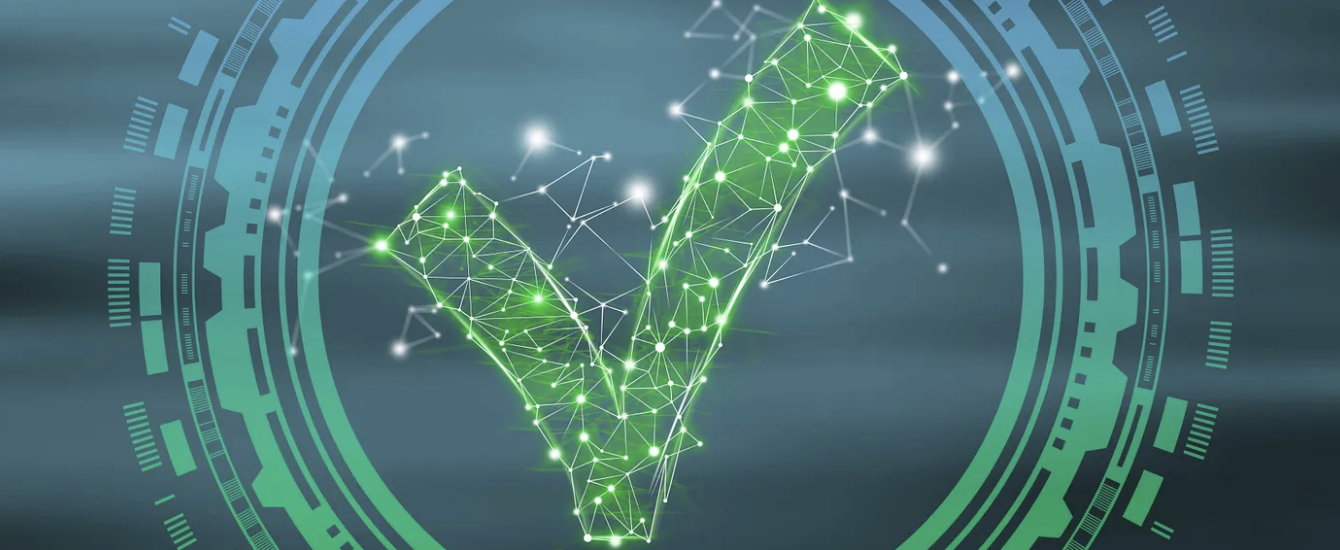